How can epidemiology models help us beat COVID-19?
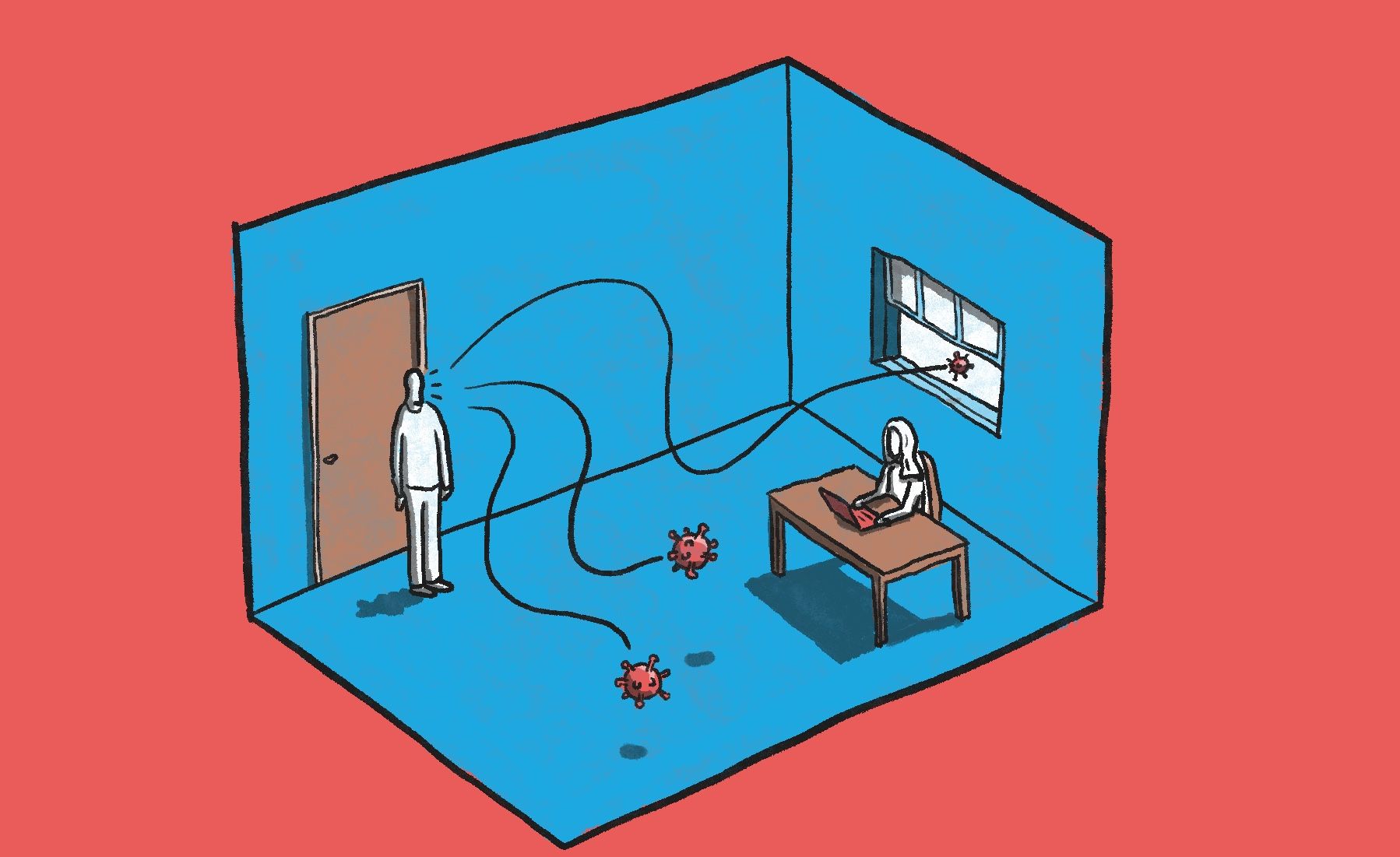
Scientists around the world have been working tirelessly to understand and reduce the spread of COVID-19. So what is an epidemiology model and how can it help us?
Epidemiology models can help us understand how COVID-19 spreads
An epidemiological model is a mathematical algorithm that describes how a disease propagates through a population in time. It takes as input information about the demographics of the population (population size, any risk factors etc), environmental factors (weather, pollution, etc), transmission probability or population mobility, and outputs a prediction of the future state of that disease in a population. The most common epidemiological model for a disease is the SEIRs model. The SEIRs model will calculate how many people in the population will be Susceptible to the disease, Exposed to the disease, Infected with the disease or Recovered from the disease. The number of people in each of these states evolves with time depending on things like: the initial state (how many people were in each category at time point zero); interventions that act against the disease (e.g. vaccines); and any other external factor that affects how the disease can progress through the population in question.
Epidemiology models play a key role in understanding and responding to the COVID-19 pandemic. But in order to build those models, scientists need to understand contributing factors and their relative importance. In this case, a large strand of literature has identified the importance of air flow to mitigate droplets and far-field aerosol transmission risks. However, the specific factors contributing to higher or lower contamination in various settings have not been clearly defined and quantified.
Non-pharmaceutical interventions (NPIs) can help reduce the spread of COVID-19
A non-pharmaceutical intervention is anything that can contribute to health that isn’t a medication. For example, while we are waiting for vaccines to be developed (a pharmaceutical intervention) NPIs could include behaviour changes we can make, or precautions we can put in place, to reduce the spread of COVID-19. Because we know that COVID-19 transmission commonly occurs in closed spaces, NPIs to address its spread should be identified and used extensively to reduce potential airborne transmissions.
The problem with modelling air flow
The modelling of airborne transmission in indoor spaces fundamentally relies on the fluid mechanics modelling of the transport of viral COVID-19 aerosols. In order to achieve accurate modelling, we must understand the contributing factors. In general, models studying airborne transmission risk fall into one of two types:
● WMR: Based on the assumption of a well-mixed room The WMR assumption is that virus-carrying aerosols are spread uniformly across the room instantaneously, so that everyone in the room, regardless of their position, is equally likely to be infected. By ignoring the complex effects of airflow on the airborne particles, this assumption greatly simplifies the problem, so the models can be incorporated into spreadsheets and are swift to run. That simplicity and lightweightness allow us to gain some insights on the COVID-19 propagation.
● CFD: Models of computational fluid dynamics CFD simulations are powerful tools to study airborne transmission, they allow for fine-grain details such as the room size, geometry, the complex turbulent airflow (e.g. open windows or HVACs) and the size distribution of the aerosols. Various CFD simulations have also investigated the transport of viral COVID-19 aerosols.
Both these types of models are only as accurate as the quality of the contributing factors considered.
Moai empowers track and trace users in the fight against COVID-19
As part of the MOAI project, we aim to identify the contributing factors systematically, proposing a simpler approach to modelling the exposure risk for all settings by taking a wholistic approach and only considering contributing risk factors coupled with proven exposures from the NHS track and trace programme.
To identify those factors, we have developed a user-based anonymous research questionnaire integrated into the MOAI app that will allow users and venues to contribute a more accurate description of the airflow in the setting. This data will be run through our ensemble model, which evaluates the likelihood of exposure of any new QR code scanning into a venue by a user.
See the full Moai research paper and all associated references here